Transforming Portfolio Management with AI: An Introductory Overview
- Nicolas Le Corre Romera
- Mar 20
- 5 min read
Updated: May 9
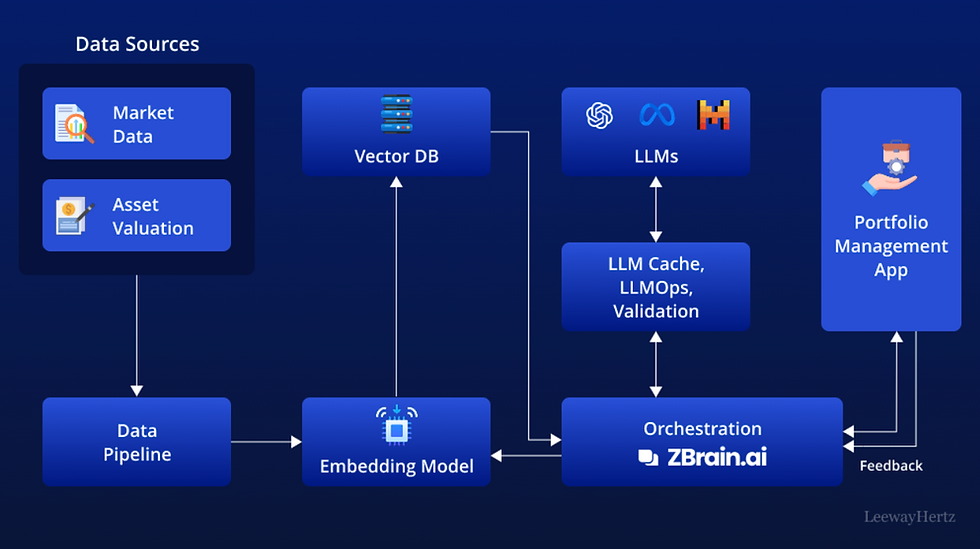
Photo from LeewayHertz
Introduction
Imagine having a financial assistant who never sleeps, continually analyzes financial markets and economic trends, and adjusts your investment portfolio in real-time to maximize your returns. That’s the promise of AI in portfolio management. As AI technology develops, financial institutions and individual investors are leveraging these advancements by gaining a competitive edge in the market and enhancing their portfolios (Lumenalta 1). Traditionally, AI was only used by large financial institutions and fintechs who had the resources to purchase large datasets to improve their models. However, with the development of robo-advisors and AI-powered investment platforms, individual investors are accessing powerful tools that were once inaccessible. (Investopedia 1, Rothschild & Co). This democratization of AI is altering how investment portfolios are being managed, making data-driven decision-making more accessible than ever. But how exactly is AI revamping the investment landscape?
AI in Portfolio Management
Portfolio Management involves selecting and overseeing a group of investments that meet an investor's objectives and risk tolerance (Investopedia 2). Traditional strategies such as Modern Portfolio Theory (MPT) and Buy and Hold have been the cornerstones of portfolio management by balancing expected return and risk. Nonetheless, these models rely on fixed assumptions, even though many factors constantly change and influence markets. AI leverages vast datasets, real-time market changes, and predictive analytics to refine investment strategies beyond traditional models (Lumenalta 1). By incorporating machine learning (ML) algorithms, reinforcement learning (RL) techniques, and sentiment analysis, AI predicts, optimizes, and measures market changes, optimizing decision-making.
Advantages of AI in Portfolio Management
One of the main advantages of AI in portfolio management is its capacity to analyze large datasets, ranging from macroeconomic trends to single stock performances, deciphering patterns, and predicting markets with greater accuracy than humans (Leeway Hertz 1). This data-driven decision-making limits the emotional aspect that can affect investment choices (FT). Moreover, risk and return forecasting can also be enhanced through AI. By using Machine Learning models, risk assessment can be optimized by evaluating asset performances under various scenarios, creating tougher portfolios that can withstand market volatility (Hyperstack).
Another critical advantage is operational efficiency. AI automation can handle repetitive and demanding tasks, including data processing, trade executions and compliance monitoring, emancipating portfolio managers to focus on innovation and strategy (Alliance Bernstein 1). By using AI to automate repetitive tasks, companies can manage more portfolios with fewer resources, reducing their costs and improving their operational efficiency. This makes AI a valuable asset not only for portfolio managers but also for large corporations.
AI Techniques Used in Portfolio Management
AI employs several advanced techniques to enhance portfolio management. Machine Learning (ML) models can predict asset price movements, identify patterns in financial data, and select high-performing stocks while adjusting to the investors' risk aversion through historical patterns (CFA Institute). These models constantly ameliorate as datasets grow, improving their accuracy over time.
Reinforcement Learning (RL) is another crucial AI used in portfolio optimization. Unlike traditional investment strategies such as MPT, which rely on fixed assumptions, RL constantly learns and adapts dynamically to market fluctuations (MDPI). RL-assisted portfolios continuously test different combinations of asset allocations, learning from their failures and improving their strategy to maximize expected returns and minimize risks. This approach is practical in highly volatile markets where quick adaptation is crucial.
Sentiment Analysis, a subfield of Natural Language Processing (NLP), plays a crucial role in AI-driven portfolio management. Sentiment analysis applies algorithms to news articles, financial data, social media, and CEO statements to gauge how people feel about the market (CFA Institute; Medium). It can detect subtle alterations in tone, word choice, and phrasing to draw conclusions about future market expectations, giving investors insights about what to expect and how they should adjust their investment portfolio accordingly.
Impact on Investors, Portfolio Managers and Financial Institutions
The growing adoption of AI in portfolio management is reshaping individual and institutional investing. Robo-advisors, a key area of financial AI, have allowed investors to access sophisticated tools, such as personalized investment strategies that consider risk aversion, financial goals, and market objectives (AmitySolutions). They can craft portfolios automatically, saving investors from a lot of the hassle and at a lower cost than human advisors (Investopedia 3).
For portfolio managers, AI improves decision-making by providing overlooked insights into market trends, automating mundane tasks, and refining risk assessment strategies (Lumenalta 2, Leeway Hertz 1). With AI handling data-intensive processes such as portfolio rebalancing, trend analysis, and trade execution, human managers can focus on high-level strategic decision-making. This symbiosis between AI and human expertise leads to more effective and efficient portfolio management.
From an institutional perspective, AI reduces costs and improves operational efficiency (Alliance Berstein 1). By analyzing large amounts of data without the need for humans, firms can reduce costs and manage larger portfolios simultaneously, improving operational efficiency. Moreover, leveraging AI reduces errors and improves regulatory compliance, making the investment management process more robust and scalable (Lumenalta 1).
Challenges and Ethical Considerations
Despite seeming like a magic solution to improve portfolios, AI brings significant challenges and ethical considerations to the table. A primary concern is explainability. Often, AI models function as "black boxes," meaning investors and regulators don't know the reasoning behind a decision or conclusion (ResearchGate 1). Understanding the reasoning behind AI allows investors to understand the functioning of the models and trust it.
Job displacement is another key challenge. For example, robo-advisors now fulfill the same duties as human advisors more efficiently and at lower costs (Research Gate 2). Despite improving efficiency and lowering costs, it causes concerns about how the job market will evolve in portfolio management. The financial industry must balance automation and human expertise to ensure a smooth transition.
Biased AI models are also a challenge. AI systems learn from historical data, which may contain biases based on past market behaviors, societal trends, or human decision-making errors (ResearchGate 2). If these biases are not identified and corrected, AI-driven portfolio strategies may inadvertently reinforce existing financial inequalities, favor certain asset classes unfairly, or overlook emerging investment opportunities. On top of that, sentiment analysis biases can influence market forecasts, resulting in erroneous decision-making. Auditing for bias and ensuring that the datasets the models are trained on are high quality and diverse is vital to preserve fairness and reliability in portfolio management.
The Future of AI in Portfolio Management
The future of AI in portfolio management will likely involve greater integration with emerging technologies such as blockchain and quantum computing (SmartDev). Blockchain can enhance transparency and security in trading and portfolio management, ensuring tamper-proof transactions and reducing fraud risks. Quantum computing could enhance portfolio optimization at unprecedented speeds and accuracy by simulating complex financial scenarios and stress-testing portfolios (Global Symposia). These advancements could further improve data security, transaction efficiency, and predictive capabilities, shaping a new era of financial strategy.
Additionally, AI-driven investment strategies are expected to become more personalized (Investopedia 1). As AI systems become more sophisticated, they will be able to tailor investment strategies to an individual’s unique financial situation, preferences, and risk tolerance. This hyper-personalization could redefine wealth management, making AI-powered financial planning more intuitive and effective than ever before.
Conclusion
To conclude, AI is revolutionizing portfolio management by making investment decisions more data-driven, efficient, and accessible. Through techniques such as Machine Learning, Reinforcement Learning,g, and Sentiment Analysis, AI improves risk management, optimizes asset allocation, and streamlines investment processes. Although challenges such as explainability, job displacement, and biases remain important to address, the pros vastly outweigh the cons. As AI continues to evolve, its role in shaping investment portfolio management will strengthen, offering new opportunities for both individual and institutional investors alike.
Comments